Introduction to Research Data Management
Introduction
With the emergence of the global Open Science (OS) movementSince the early 2000s, the Open Science movement has advocated for an open and transparent approach to science in which all stages of the scientific knowledge process are made openly accessible online. This means that not only the final results of research, such as monographs or articles, are shared publicly, but also materials that accompanied the research process, such as lab notebooks, research data, software used, and research reports. This approach aims to promote public participation in science and knowledge, engaging interested audiences. It also seeks to encourage creativity, innovation, and new collaborations, while enabling the verification of findings in terms of quality, accuracy, and authenticity – a process intended to democratize research. Components of Open Science include Open Access and Open Data, which provide the infrastructure for sharing interim research results. Read More in the early 2000s, expectations for responsible research and good scientific practiceGood scientific practice (GSP) represents a standardized code of conduct established in the guidelines of the German Research Foundation (DFG). These guidelines emphasize the ethical obligation of every researcher to act responsibly, honestly, and respectfully, also in order to strengthen public trust in research and science. They serve as a framework for guiding scientific work processes. Read More (GWP) have evolved. GWP constitutes a standardized code embedded in the guidelines of the German Research Foundation (DFG) and commits researchers to honest, responsible, and ethically and legally sound scientific work (DFG, 2022). Increasing emphasis is also placed on demands for Open AccessOpen Access refers to the free, costless, unrestricted, and barrier-free access to scientific knowledge and materials. For third parties to reuse these materials legally, the creators must grant usage rights through a licensing agreement. Free CC licenses, for example, specify exactly how data and materials may be reused. Read More – free and unrestricted access to research data – and consequently Open DataOpen data are data that are openly and freely accessible online and may be reused by third parties without restriction. This requires that they are provided with an open license (Opendefinition, 2023). Read More, enabling the reuse of data by third parties. Open Science principles are enshrined in DFG guidelines and recommendations, aiming to make academic research accessible to diverse publics, strengthen trust in science, and foster creativity, innovation, and collaboration.
According to the FAIR principlesThe FAIR Principles were first developed in 2016 by the FORCE11 community (The Future of Research Communication and e-Scholarship). FORCE11 is a community of researchers, librarians, archivists, publishers, and research funders aiming to bring about change in modern scientific communication through the effective use of information technology, thereby supporting enhanced knowledge creation and dissemination. The primary goal is the transparent and open presentation of scientific processes. Accordingly, data should be made findable, accessible, interoperable, and reusable (FAIR) online. The objective is to preserve data long-term and make it available for reuse by third parties in line with Open Science and Data Sharing principles. Precise definitions by FORCE11 can be found on their website see: https://force11.org/info/the-fair-data-principles/. Read More, first published by the FORCE11 community (The Future of Research Communication and e-Scholarship) in 2016, scientific findings should circulate transparently and openly even during their development (Force, 2021). Data should be findable, accessible, interoperableInteroperability is the ability of a system to work seamlessly with other systems. In interoperable systems, data can be automatically combined and exchanged with other datasets, making data machine-readable, interpretable, and comparable in a simplified and accelerated manner. Interoperability is one of the main criteria of the FAIR Principles (Forschungsdaten.info, 2023). Read More, and reusable - structured, documented, and stored accordingly. Unrestricted access to knowledge is intended to enable broader participation in academic discourse and promote the democratization of research.
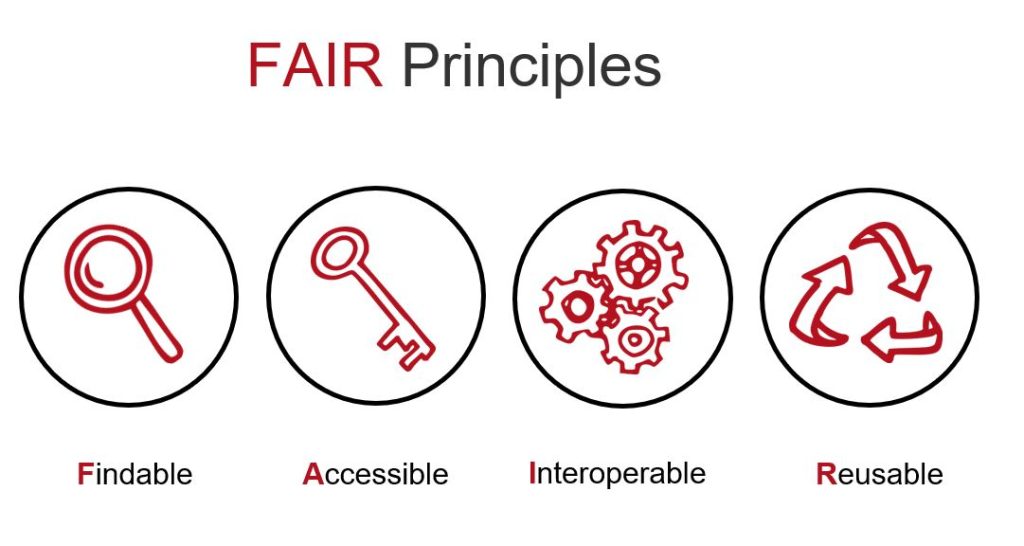
Source: FAIR Principles (based on Paulina Halina Sieminska), Anne Voigt with CoCoMaterial, 2023, licensed under CC BY-SA 4.0
A core focus in this context is research data management (RDM). RDM is a key concept of responsible and good scientific practice and encompasses the handling of research data concerning its organization, maintenance, and processing through specific measures and strategies. The goal is to preserve data in the long term in accordance with the FAIR principles and make it accessible to third parties so that scientific claims can be verified, evidence secured, and further evaluations or analyses conducted. This aligns with the imperative of Data SharingData sharing refers to the act of sharing or distributing data. According to research requirements, data should be made as open as possible and as confidential as necessary (European Commission, 2021). Particularly with regard to the reuse and handling of sensitive, personal data, it is crucial to carefully assess whether and in what form archiving and sharing data with other researchers and the public is possible and appropriate. The imperative of data sharing enjoys broad consensus within the Open Science movement but should be critically considered and weighed from a social and cultural anthropological perspective. Read More – the sharing and dissemination of data. According to Open Science'Open Science encompasses strategies and practices aimed at making all components of the scientific process openly accessible and reusable on the internet. This approach is intended to open up new possibilities for science, society, and industry in handling scientific knowledge” (AG Open Science, 2014, translation by Saskia Köbschall). Read More principles, research data should be presented and made available "as open as possible and as closed as necessary" (European Commission, 2021).
In recent years, research data management has gained increasing importance, leading more universities, research institutions, and funding bodies to establish their own research data policies. These policies and guidelines provide support on RDM-related questions and should be considered in implementation. While Germany does not yet have standardized regulations for handling research data, funding programs from the DFG or the EU may require compliance with specific documentation, such as a data management planA data management plan (DMP) describes and documents the handling of research data and materials during and after the project period. The DMP specifies how data and materials are generated, processed, stored, organized, published, archived, and, if applicable, shared. Additionally, it outlines responsibilities and rights. As a 'living document' (a dynamic document that is continuously revised and updated), the DMP is regularly reviewed and adjusted as needed throughout the course of the project. Read More.
A recommended structured approach to RDM is provided by the Research Data LifecycleThe research data lifecycle model represents all the phases that research data can go through, from the point of collection to their reuse. These phases are linked to specific tasks and may vary (Forschungsdaten.info, 2023). Generally, the research data lifecycle includes the following stages: Read More model, which serves as a practical tool. This model categorizes the different "life stages" of data and associates them with specific tasks that arise before, during, and after data collection. These include research planning, data collection, data processing and analysis, data publication, archiving, and reuse. The lifecycle metaphor highlights data as "living" entities that continue to have relevance beyond the original research project, potentially through reuseData reuse, often referred to as secondary use, involves re-examining previously collected and published research datasets with the aim of gaining new insights, potentially from a different or fresh perspective. Preparing research data for reuse requires significantly more effort in terms of anonymization, preparation, and documentation than simple archiving for storage purposes. Read More.
The FAIR principles, university guidelines, and the research data lifecycle offer recommendations for successful research data management but only marginally address ethical aspectsResearch ethics addresses the relationship between researchers, the research field, and the subjects/participants of the research. This relationship is critically examined against the backdrop of vulnerabilities and power asymmetries created by the research process (Unger, Narimani & M’Bayo, 2014, p.1-2). Due to the processual and open-ended nature of ethnographic research, ethical questions arise throughout the research process in various ways, depending on the research context and methods. However, research ethics does not end with leaving the field; it also encompasses issues related to data archiving, data protection, and sharing research data with participants (see, for example, ethics guidelines by the DGSKA or the position paper on archiving, provision, and reuse of research data by the dgv). Read More and challenges. To complement these principles, the Research Data Alliance – which aims to expand technical and social infrastructures for data sharing – introduced the CARE principlesThe CARE principles were established by the Global Indigenous Data Alliance (GIDA) in 2019. They complement the FAIR principles and are used as a tool to focus more strongly on research contexts and their historical embeddedness, as well as on power asymmetries in the field. The acronym stands for Collective Benefit (common good), Authority to Control (control of research participants over their own representation), Responsibility (responsibility on the part of researchers) and Ethics (consideration of ethical aspects). The CARE principles are intended to emphasize and take into account the fair, respectful and ethical treatment of research participants and the data generated from research with regard to data sharing. The CARE principles are therefore relevant in all phases of the research data life cycle and research data management. Read More in 2019. These are particularly relevant for social and cultural anthropological research (see article on research ethics; Research Data Alliance, 2016).
Motivation
Several factors motivate the implementation of research data management:
- Certain funding institutions require RDM strategies (such as a data management plan) as a prerequisite for funding (see article on data management plans).
- By adhering to RDM processes from the outset, researchers not only facilitate their own future reinterpretation of their data but also reduce the effort required to prepare data for reuse by third parties.
- Reproducibility and traceability of research findings depend on well-documented RDM practices (RatSWD, 2023, p. 8).
- The risk of data loss is minimized through RDM measures such as data protectionData protection includes measures against the unlawful collection, storage, sharing, and reuse of personal data. It is based on the right of individuals to self-determination regarding the handling of their data and is anchored in the General Data Protection Regulation (GDPR), the Federal Data Protection Act (Bundesdatenschutzgesetz), and the corresponding laws of the federal states. A violation of data protection regulations can lead to criminal consequences. Read More, data securityData security encompasses all preventive physical and technical measures aimed at protecting both digital and analog data. Data security ensures data availability and safeguards the confidentiality and integrity of the data. Examples of security measures include password protection for devices and online platforms, encryption for software (e.g., emails) and hardware, firewalls, regular software updates, and secure deletion of files. Read More, systematic documentation, and long-term archiving (LZA).
- Good RDM practices support the implementation of the FAIR principles.
However, research data management does not necessarily mean that data must be openly accessible. There may be legal (e.g., usage rights) or ethical reasons against Open Science that must be carefully considered.
Methods
The measures and strategies of research data management (RDM) encompass the following areas, which are explored in more detail in subsequent articles (see also the guide that thematically clusters the articles):
- Planning the research project and data generation (fieldwork), as well as creating a data management plan (DMP)
- (Digital) recording strategies
- Measures to protect personal data
- Processing, evaluation, and analysis of data
- Data documentation
- Data publication
- Long-term archiving and reuse scenarios
Practical Examples
Example: Interview of Birgitt Röttger-Rössler with Max Kramer (2023)
In this interview, social and cultural anthropologist Dr. Max Kramer discusses his current research on Muslim minorities in India and their media practices, specifically the tactical use of digital platforms by activist
As audio file, only in German
Audio Source: Interview Röttger-Rössler with Kramer on Research in India, 2023, licensed under CC BY-NC-ND 4.0
As transcript
Birgitt Röttger-Rössler: I am speaking with social anthropologist Dr. Max Kramer, who focuses on religious minorities in India, particularly Muslim communities and their media practices. Max, if I understood correctly, you are primarily interested in how religious minorities tactically use digital platforms. That is, you examine how activists’ experiences in their analog lives are represented online.
Max Kramer: Well, that’s not entirely accurate – primarily because these activists no longer have purely "analog" lives. Their everyday world is deeply mediatized, meaning that there is no meaningful separation between online and offline. Instead, they strategically utilize various platform affordances, which present both opportunities and risks. I understand tactics as something that emerges from long-term learning, and this learning is not solely about representation. My main interest is in what one could call ethical questions – how to emotionally prepare for Twitter engagement, why one sometimes chooses to write a poem instead of posting a politically charged tweet, or why one may withdraw from social media for months to observe political opponents and study India's racialized digital ecosystem, largely built by Hindu nationalists over the past 15 years.
One develops a sense of when and how to post the right content, on the right platform, with the right emotions. This tactical refinement is acquired through a sometimes brutal learning process. Otherwise, one may be 'framed' or used as an instrument in the staging of moral outrage by political opponents. My interlocutors carefully consider what they can do to prevent this from happening.
Birgitt Röttger-Rössler: If I may briefly interject – this is very interesting, what you’re describing - because you have convincingly shown us that the separation between online and offline, between the virtual or digital and the analog world, can no longer really be maintained. That all these media and digital practices shape our everyday lives, and that this separation has essentially become artificial. This, of course, also presents a challenge for social anthropology – we have to find ways to deal with it. So, how do you approach this? You mentioned to me in our previous conversations that you initially followed the leading political activists – those with significant influence, large Twitter handles, or, as we should now say, X handles – online and tracked what they were doing, how they represented themselves, whom they interacted with, and whom they responded to. And then you tried, of course, to establish contact with these people through your many existing connections. So, how did you document and store your online research? That’s something I’d like to know. And also, how do you document this entanglement between the analog and digital worlds that you just spoke about?
Max Kramer: It’s more of a circular process. I already had networks in Delhi and Mumbai from previous research, and I had to read and retweet content related to these actors – who were usually people with relatively large handles. By that, I mean more than 50,000 followers, sometimes even up to 150,000 followers – perhaps one could call them Twitter micro-star personas. I initially followed all of them. There aren’t many individuals across India who have such a large following. And I then tried to meet these people in person as quickly as possible. So, my primary data consists of conversations about practice. What interests me is what matters to these actors – what is important to them in their practice? What problems do they face when using social networks, and how do they learn to deal with these problems, avoid them, and develop new tactical approaches? Before my first meeting with activists, I would usually review their tweets from the past few months and occasionally take screenshots if I thought a particular tweet had been widely shared, was heavily debated, or had even led to a lawsuit against these individuals. These were the tweets I took screenshots of and then brought into the first conversation. However, I soon realized that the screenshots I had taken beforehand were not necessarily of tweets that were important to the activists themselves. The tweets that I had selected were often insignificant to their own memory of their Twitter history, while entirely different tweets held much greater significance for them. So I then began engaging with these tweets, taking screenshots of them, organizing them into folders, encrypting those folders, and storing them securely on an encrypted hard drive. That’s what I do with all the data I collect.
Birgitt Röttger-Rössler: That brings us to a very, very important question. In your research, the particular challenge is its political sensitivity – Muslim minority groups, as you’ve already mentioned, are repeatedly subjected to hostilities from Hindu nationalists. How do you deal with that? You’ve already hinted at it, but perhaps you could add a bit more. How do you ensure the safety of your interlocutors? And to what extent does this affect – or rather, influence – how you handle your research data?
Max Kramer: In every possible way. For example, in the field, we don’t use WhatsApp but Signal for communication. When I’m in the field, I set Signal to automatically delete messages after 30 minutes. I make sure that whatever I record as raw data is secure – securely recorded, securely stored, and securely processed. For recording videos, photos, and audio files, I use a separate phone in the field – one without a SIM card, running GrapheneOS, a secure operating system. This phone also has encryption software, which I use every evening to encrypt all the files I recorded during the day.
And in my book – so this is all at the level of data collection, transport, storage, processing, and especially transcription – which is the most sensitive aspect of my research. Because people are giving me insight into their operational knowledge, and there is information included that – although these individuals are, in a way, micro-stars and quite visible – the specific knowledge I have is not visible and must never end up in the wrong hands. This also means that I have to carefully consider how I process my data and how I present it in my arguments. And I experiment a bit – not only with anonymization but also with fictionalization. By that, I mean that some locations and events need to be shuffled or altered as long as the general context remains intact – just enough to support the argument. Otherwise, these individuals could be too easily tracked. For example, I can’t simply copy and paste tweets into my finished text - that would immediately expose any attempt at anonymization. So, any data that is easily traceable, that could be linked to a person with just a few clicks, cannot be reproduced in its original form. Instead, tweets must be paraphrased, so that the context remains, but nothing can be traced back to the original source. Now, this creates an interesting tension, because the people I work with - these micro-stars - are political figures, and they receive a lot of recognition for their courage. Some of them are also poets, and their aesthetic refinement should be acknowledged. Moreover, many of them explicitly tell me that they want to be named. So, this is also a difficult trade-off for me.
Birgitt Röttger-Rössler: Yes, I think that is a completely different and much greater challenge – how do you represent this material, given the tension you just described? Some of our interlocutors want to be named – they do not want to disappear behind anonymization. And yes, that is a major challenge.
Max, I really appreciate this conversation. I think you have raised many, many important points, particularly regarding the level of thought that must go into encryption and secure data storage in the field. In my experience, many researchers still approach this issue far too naively. So, thank you very much for this conversation.
Literature and References
Deutsche Forschungsgemeinschaft. (DFG, 2022). Leitlinien zur Sicherung guter wissenschaftlicher Praxis. Kodex. https://doi.org/10.5281/zenodo.6472827
Europäische Kommission, Directorate-General for Research and Innovation. (2021). Horizon Europe, open science. Early knowledge and data sharing, and open collaboration. Publications Office of the European Union. https://data.europa.eu/doi/10.2777/18252
Force11. (2021). The FAIR Data Principles. Force11. The Future of Research Communications and e-scholarship. https://force11.org/info/the-fair-data-principles/
Rat für Sozial- und Wirtschaftsdaten. (RatSWD, 2023). Forschungsdatenmanagement in kleinen Forschungsprojekten – Eine Handreichung für die Praxis. RatSWD Output Series, 7. Berufungsperiode Nr. 3. https://doi.org/10.17620/02671.72
Research Data Alliance. (RDA, 2016). The Research Data Alliance (RDA) builds the social and technical bridges to enable the open sharing and re-use of data. Research Data Alliance. https://www.rd-alliance.org/about-rda
Sieminska, P. H. (2019). A FAIRy tale graphics (1.0.0). Zenodo. https://doi.org/10.5281/zenodo.3267168
Additional Literature
Deutsche Gesellschaft für Sozial- und Kulturanthropologie. (DGSKA, 2015). Positionspapier zum Umgang mit ethnologischen Forschungsdaten. Forschungsdaten Info. https://forschungsdaten.info/nachrichten/nachricht-anzeige/positionspapier-zum-umgang-mit-ethnologischen-forschungsdaten/
Forschungsdaten.Info. (2022). Forschungsdaten und Forschungsdatenmanagement: Richtlinien und Policies: Wozu werden diese benötigt? Forschungsdaten.Info. https://forschungsdaten.info/themen/ethik-und-gute-wissenschaftliche-praxis/leitlinien-und-policies/
Forschungsdaten.info. (2023f). Was ist Forschungsdatenmanagement?. forschungsdaten.info. https://forschungsdaten.info/themen/informieren-und-planen/was-ist-forschungsdatenmanagement/
Reilly, M. & Thompson, S. (2020). Understanding Data Management Planning and Sharing: Perspectives for the Social Scientist. In Crowder, J. W., M. Fortun, R. Besara, L. Poirier. (Eds.) Anthropological Data in the Digital Age (13–30). Palgrave Macmillan Cham. https://doi.org/10.1007/978-3-030-24925-0
Citation
Heldt, C. & Röttger-Rössler, B. (2023). Introduction to Research Data Management. In Data Affairs. Data Management in Ethnographic Research. SFB 1171 and Center for Digital Systems, Freie Universität Berlin. https://en.data-affairs.affective-societies.de/article/introduction-to-research-data-management/